Harnessing AI and Credit Data to Boost Acquisition Win-Rates in Prescreen Marketing
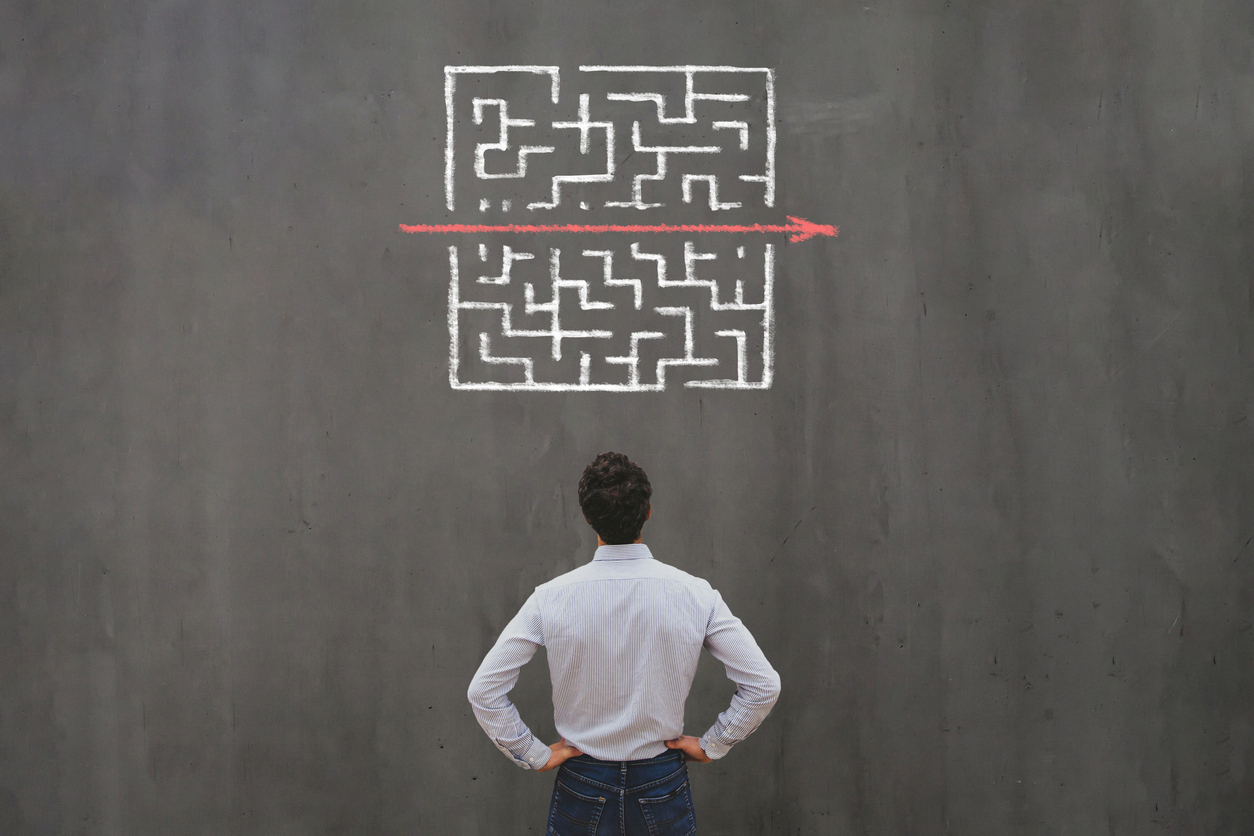
By Devon Kinkead
The difference between a profitable and unprofitable acquisition campaign often comes down to data intelligence. Prescreened credit offers remain one of the most powerful tools for acquiring new customers, but many institutions are still shooting in the dark. The convergence of artificial intelligence and rich credit data is revolutionizing how financial institutions can systematically improve their conversion rates and win rates.
The Challenge: Turning Lost Opportunities into Wins
Financial institutions face a common frustration: sending thousands or millions of prescreen offers only to see disappointing conversion rates. Take a recent auto loan refinance campaign we analyzed:
- 9,845 offers were distributed
- 8 loans acquired (0.08% conversion rate)
- 398 customers chose competitors (4.12% total conversion)
- 1.97% win-rate in the prescreen list (8 loans won/(398 loans lost +8 loans won))
- Break-even return on investment
These numbers reveal millions in lost revenue opportunities and thousands of potential accountholder relationships that never materialized.
The AI-Powered Approach to Prescreen Marketing
Here’s how forward-thinking financial institutions are using AI and credit data to transform their acquisition strategies:
1. Pattern Recognition Beyond Human Capability
Traditional analysis might segment customers by basic credit score bands or geographic regions. AI systems, however, can identify complex patterns across hundreds of variables simultaneously. These systems can detect subtle correlations between:
- Credit score fluctuation patterns over time
- Specific combinations of credit utilization and debt-to-income ratios
- Geographic and competitive influences on rate sensitivity
- Loan characteristic preferences based on past borrowing behavior
By analyzing actual win/loss data from previous campaigns, AI can identify which specific factors influenced a prospect’s decision to accept or reject offers—insights that would be impossible to discern through conventional analysis.
2. Predictive Modeling with Back-Testing
The true power of AI in prescreen marketing lies in its predictive capabilities combined with back-testing for human review:
- Predictive Targeting: AI can predict which prospects are most likely to respond positively to specific offer terms.
- Counter-Factual Analysis: For each lost sale, AI can model “what if” scenarios to determine which adjusted offer terms would have improved the odds of winning a particular customer and why.
- Strategy Simulation: Before launching a modified campaign, AI can simulate expected results based on historical response patterns.
In a recent analysis, we used AI to identify three strategic adjustments to an auto refinance campaign. Our models predicted these changes could increase the win rate from 1.97% to 6.00%—more than tripling the campaign’s win-rate and corresponding lender competitiveness.
3. From Broad Segments to Individual-Level Personalization
Traditional prescreen campaigns operate at the segment level—everyone in a particular credit band receives roughly the same offer. AI enables a shift toward truly individualized offers while remaining compliant with FCRA/UDAAP regulations and fair lending laws.
Real-World Strategy Development: A Case Study
To illustrate the power of this approach, consider how AI can transforms a lender’s auto refinance strategy:
- Data Integration: We combined the lender’s prescreen campaign data with detailed information on lost sales, including which sales were lost at what terms.
- Pattern Discovery: AI analysis revealed three critical insights:
- High-FICO borrowers (700+) were extremely sensitive to rate differences as small as 0.5%
- Large loans (>$30,000) had materially different success factors than smaller loans
- Certain geographic markets showed unique competitive dynamics requiring tailored approaches
- Strategy Development: Based on these insights, the AI recommended three specific strategies:
- Tiered rate adjustments for high-FICO borrowers
- A specialized fast-track program for loans over $30,000
- Geographic-specific incentive bundles for high-competition markets
- Back-Testing Validation: Before implementation, each strategy was back-tested against historical data, confirming that these approaches would have converted more specific lost opportunities into wins.
- Implementation Roadmap: The final output included a detailed implementation plan with projected ROI for each strategy component.
Back-Testing Results: Turning Theory into Wins
The true power of AI-driven strategy development is the ability to back-test recommendations against actual prospect data. Below are 9 examples from the lender’s lost sales data that demonstrate exactly how each proposed strategy would have improved the odds of converting specific lost sales into wins:
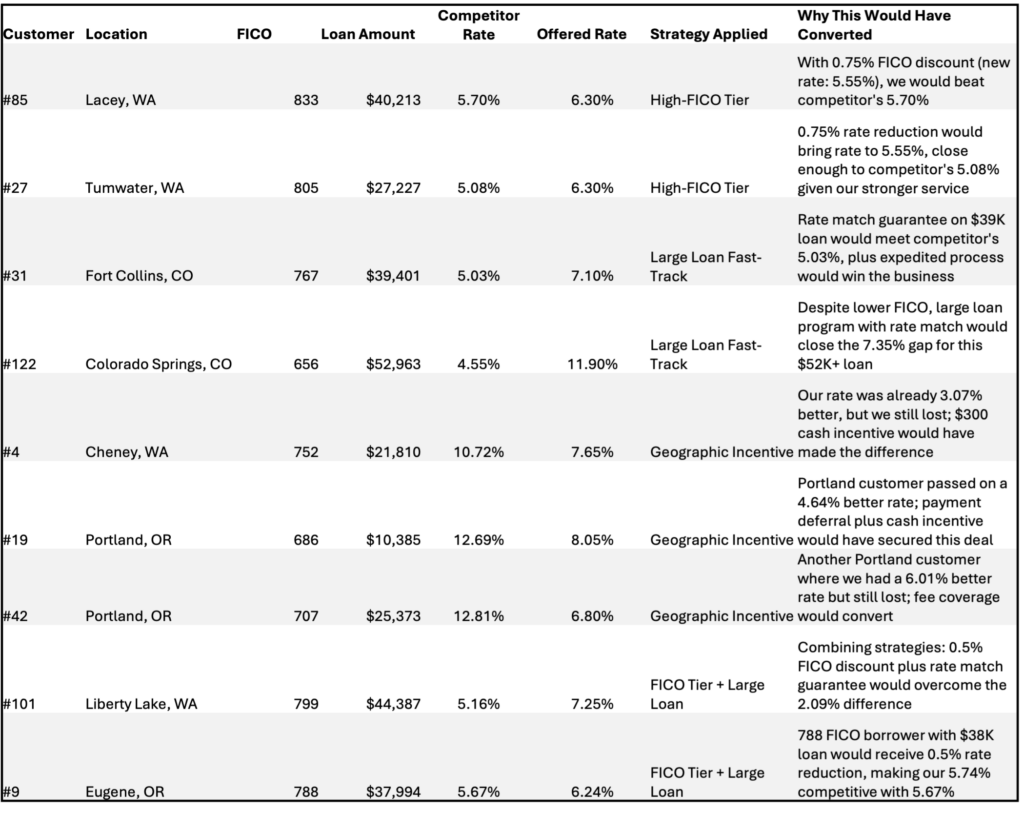
This table isn’t theoretical—it’s built from actual loss data, showing precisely which lost prospects would likely have been converted with the recommended strategies. The power lies in the specificity and explainability: we can point to exact customer profiles and competitor offers that would have resulted in different outcomes had these strategies been in place.
Moving Beyond Intuition to Data-Driven Certainty
The most significant shift in this AI-powered approach is moving from intuition-based marketing to data-validated and back-tested strategies. Every recommendation is backed by concrete examples from your own prospect portfolio—specific customers who would have a higher probability of converting with the proposed changes.
This approach doesn’t just drive higher conversion rates; it creates a continuous learning system where each campaign becomes smarter than the last. Your marketing doesn’t just improve incrementally—it evolves strategically even if every recommendation isn’t immediately implemented.
The Future of Prescreen Marketing
As AI systems become more sophisticated and regulatory frameworks evolve, we’re moving toward an agentic future with:
- Real-Time Offer Optimization: Adjusting offer terms dynamically as market conditions shift.
- Cross-Product Intelligence: Using insights from one product line to enhance targeting in others.
- Regulatory Compliance Automation: Ensuring all personalized offers meet FCRA/UDAAP and fair lending requirements.
- Behavioral Economics Automation: Ensuring that offers are optimized for the way people make choices.
Getting Started with AI-Powered Prescreen Marketing
For financial institutions looking to harness these capabilities, the journey begins with asking better questions of your data:
- Don’t just measure campaign success—analyze your failures at an individual level
- Capture and integrate competitive intelligence on lost opportunities
- Look beyond basic credit metrics to multidimensional patterns
- Invest in back-testing capabilities to validate strategies with humans before deployment
- Build a continuous learning loop between campaigns
The financial institutions that thrive in the coming decade won’t just be those with the largest marketing budgets—they’ll be the ones that use AI and credit data most intelligently to identify and convert the right prospects with the right offers at the right time.
In a world where basis points of market share translate to millions in revenue, the competitive edge gained through AI-powered prescreen marketing isn’t just valuable—it’s essential. Talk to Micronotes today about the future of prescreen marketing.